Combining Curated Information & AI to Help Solve Information Overload
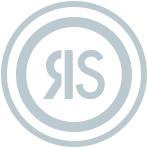
AI is clearly at the forefront of everyone’s mind when it comes to influential and transformative trends in business. Across industries, this technological advancement's implications and potential applications are being hotly discussed and debated.
Scientific research and knowledge discovery is no exception.
Therefore, we asked our community about their experience level and their thoughts about the AI landscape.
The results are in:
There is a lot of excitement around the technology, with some experience, but not significant usage. At varying levels of adoption, but with a desire to solve pressing problems using AI, it’s worth taking some time to examine various examples and potential solutions, looking at different use cases in research.
Which Problems Are We Solving With AI?
When thinking about solutions, carefully considering the challenge or problem you are solving is essential. In this case, broadly, it's the challenge of information overload.
So, in thinking about how to define this challenge, we did what many of us are increasingly doing these days: we turned to ChatGPT to help summarize what information overload potentially means.
It says:
Illustrating this particular definition of information overload is the exponential growth of research articles and content.
Exponential Growth of Scientific Research Articles Over the Last 200 Years*
We are publishing more and more as time goes on: critical, peer-reviewed information that academic and corporate researchers need to access, analyze, and extract data from to aid in their own research. Scientific and corporate research encompasses various data types beyond scientific journal articles, including:
- Patents
- Clinical Trial Data
- Grant Funding
- Genomics Data
- Experimental Data
- Competitive Intelligence
- Social Media
- News
- Policy Documents
- And Much More
Critically, the research process is more than simply finding specific pieces of information or that one critical article needed for your current research; you must also understand the connections between topics and concepts, as well as between authors and collaborators. This is important because it is understanding these very connections that advances research.
Helping Or Hurting? Generative AI In Research
Having defined the present challenge, we address the topic of Generative AI (such as ChatGPT) and its role as part of the solution, and contribution, to the information overload problem.
Having surpassed one million users within one week of its launch, it’s safe to say that most of us have used Generative AI to some degree by this point. In the next year or two, it will significantly improve the way scientific researchers are able to tackle such processes as:
- Creating journal articles from their research;
- Producing successful manuscripts; and
- Expediting the publication process.
While, in these ways, Generative AI is clearly a part of the overall solution, we predict that the information overload issue will gain velocity in the upcoming year because of it.
Using AI To Build Knowledge Discovery Systems
Since AI is a very broad term, the types of AI technologies we are examining here are specifically Natural Language Processing (NLP) and Machine Learning (ML), which includes deep learning and neural networks, and closely related to that are Large Language Models (LLMs), such as Generative AI.
There are many ways AI is used to create Knowledge Discovery Systems to address various use cases, such as Content Discovery, Trend Analysis, Prediction, and Hypothesis Generation.
It’s important to think holistically about Knowledge Discovery Systems. It's more than just the end solution, how you interact with information and get it back in different forms, and the specific use case being addressed. It’s also about leveraging the key foundational components of proficient Knowledge Discovery Systems, including Taxonomies, Ontologies, and Knowledge Graphs, and how we can further use NLP, ML, and LLMs to increase usability, efficiency, and accuracy.
Best of Both Worlds Combining Curated Information Frameworks with Generative AI
The debate around the utility of AI systems and, more recently, Generative AI systems like ChatGPT invariably highlights the limitations and risks of relying on the outputs, which can be inaccurate and biased.
Foundational components of proficient Knowledge Discovery Systems, including Taxonomies, Ontologies, and Knowledge Graphs, have a critical part to play in enabling AI systems to provide more transparency on the sources of information being surfaced in the output.
Taxonomy
Taxonomies provide a systematic framework for organizing and categorizing diverse elements. They are used in fields such as linguistics, library science, information technology, and business. They help in information retrieval, data organization, knowledge management, and facilitating effective communication and understanding.
Ontology
An ontology is also a formal and explicit representation of the concepts, entities, and relationships within a particular domain of knowledge with a hierarchical structure. Ontologies are more expressive than Taxonomies and provide a richer representation of knowledge. Ontologies can capture complex relationships, constraints, and rules within a domain, making them suitable for advanced reasoning and inference.
Knowledge Graph
A Knowledge Graph is a type of knowledge representation that stores and organizes structured data in a graph database. In a knowledge graph, entities (such as objects, concepts, or individuals) are represented as nodes, and their relationships are represented as edges connecting these nodes. Each node and edge typically has associated attributes or properties that provide information about the entities and relationships.
By using Ontologies and Taxonomies, Knowledge Graphs can establish meaningful relationships between entities from different datasets (sources) or domains.
Some use cases include:
- Trend Analysis: These frameworks help researchers identify, explore, and accurately track research trends over time. Topics can be seen first appearing in conference proceedings, becoming the focus of an increasing number of researchers and collaborations. This then leads to scientific journal publications where the topic is picked up by industry as applied research finds novel innovations based on that topic, resulting in patents and new products and technologies.
- Prediction: Once we can analyze trends, we can go further with modeling and build machine learning systems to look at predicting future trends by considering a variety of relationships between concepts and entities. In this way, we are able to forecast the areas and topics that will have the greatest impact on future research. This kind of intelligence is extremely valuable, not only to commercial industries and academia, but also to governments, funding organizations, and regulatory bodies.
- Hypothesis Generation: Increasingly, Knowledge Graphs, combined with ML (Deep Learning), are used to help define hypotheses to be tested. For instance, in Drug Discovery, Knowledge Graphs based on life science literature have successfully been applied to shortlist existing drugs that could be re-purposed as new therapies.
- Experimental Design: By leveraging LLMs, such as ChatGPT, researchers can then define the experimental protocols that could be used to test the hypotheses.
- Transparency & Accuracy: Retrieval Augmented Generation (RAG) refers to the focus of LLMs on curated data sets or Knowledge Bases such as Knowledge Graphs. RAG takes advantage of the "Generative" abilities of LLMs while ensuring inaccuracies and "Hallucinations" are avoided by clearly referencing and linking to the sources of the facts and information included in the generated texts.
Information overload is the defining challenge and opportunity of scientific research. A challenge where foundational knowledge frameworks, such as Taxonomies, Ontologies, and Knowledge Graphs, are created and combined with NLP, ML, and LLM technologies uniquely suited to providing solutions. Solutions that are set to significantly increase the pace and breadth of scientific discovery and innovation.
Discover The Latest Breakthroughs in Research & Stay Ahead in Your Field
Accelerate your research with the power of AI. At Research Solutions, we are dedicated to helping you reach new heights and gain results faster. By incorporating AI features into our products, we enable organizations to streamline and scale their research processes. Our focus is on creating innovative search and discovery experiences using breakthrough NLP models like ChatGPT. Imagine the potential these advanced tools hold for your research.
Uncover new possibilities with Research Solutions. Don't miss out, book a demo today!
*Source: Dimensions